![]() |
Sohail Bahmani |
Research Vignette
Let \(X\in \mathbb{R}^d\) be a random vector. Given a norm \(\left\|\cdot\right\|\) defined over \(\mathbb R^d\), a problem of fundamental interest is finding tail/moment bounds for \(\left\| X\right\|\). This problem is studied in various forms and level of generality in functional analysis, probability theory, and statistics. The standard firs step is to express \(\left\| X\right\|\) as the supremum of linear forms index by \(\mathcal B_*\), the unit ball of the dual norm \(\left\|\cdot\right\|_*\), as \[\left\| X\right\| = \sup_{u\in \mathcal B_*}\left\langle u,X\right\rangle\,.\nonumber\] Then, we can approximate \(\mathcal B_*\) by a suitable finite set \(\widehat{\mathcal B}_*\) and apply union bound to obtain a tail/moment bound from the tail probabilities or moments of \(\left\langle u,X\right\rangle\) for \(u\in \widehat{\mathcal B}_*\). The chaining method, and it's more refined version, Talagrand's method of generic chaining follow the mentioned basic approximation idea but optimize the choice of the approximation set \(\widehat{\mathcal B}_*\) and use the union bound in a more efficient way. In particular, for Gaussian processes (i.e., \(X\) being Gaussian), Talagrand's majorizing measures theorem shows that the generic chaining method captures the size of \(\mathbb E\left\| X\right\|\) up to constant factors.
The family of approaches mentioned above are all fundamentally based on the idea of inner approximation of the index set. However, we can approximate the index set from the outside as well. For example, for a sufficiently large positive integer \(N\), let \(u_1,\dotsc, u_N\in \mathbb R^d\) be such that their absolute convex hull contains \(\mathcal B_*\), i.e., \(\mathcal B_* \subseteq \mathrm{absconv}\left\{u_1,\dotsc,u_N\right\}\). Then, for any \(p\ge 1\) and every \(x\in \mathbb R^d\) we can write \[ \left\| x\right\|^p \le \max_{i=1,\dotsc,N} \left|\left\langle u_i, x\right\rangle\right|^p \le \sum_{i=1}^N \left|\left\langle u_i, x\right\rangle\right|^p\,. \nonumber \] This commonly used elementary inequality can be refined, by observing that the sum on the right-hand can be normalized by the number of term for which \(\left|\left\langle u_i, x\right\rangle\right| \ge \left\| x\right\|\). In particular, we have \[ \left\| x\right\|^p \le \frac{\sum_{i=1}^N \left|\left\langle u_i, x\right\rangle\right|^p}{\sum_{i=1}^N 𝟙\left(\left|\left\langle u_i, x\right\rangle\right| \ge \left\| x\right\|\right)}\,. \nonumber \] It then follows that \[ \left\| X\right\|^p \le \frac{\sum_{i=1}^N \left|\left\langle u_i, X\right\rangle\right|^p}{\inf_{x\in \partial \mathcal B}\sum_{i=1}^N 𝟙\left(\left|\left\langle u_i, x\right\rangle\right| \ge 1\right)}\,, \nonumber \] where \(\partial \mathcal B\) denotes the boundary of the unit ball of \(\left\|\cdot\right\|\). We can express this inequality in a more general probabilistic form, namely for a random variable \(U\in \mathbb R^d\) whose distribution is \(\mathbb{P}_0\) and is independent of \(X\) we have \[ \left\| X\right\|^p \le \frac{\mathbb{E}_0 \left( \left|\left\langle U, X\right\rangle\right|^p\right)}{\inf_{x\in \partial \mathcal B} \mathbb{P}_0\left(\left|\left\langle U, x\right\rangle\right| \ge 1\right)}\,, \nonumber \] with \(\mathbb{E}_0\) denoting the expectation with respect to \(U\). Then taking expectation with respect to \(X\) on both sides we obtain a bound for \(\mathbb{E} \left\|X\right\|^p\) which can also be converted to a tail bound using Markov's inequality. Of course we can optimize the bound with respect to \(\mathbb P_0\) and deduce \[ \mathbb E\left\| X\right\|^p \le \inf_{\mathbb P_0} \sup_{x\in \partial \mathcal B} \frac{\mathbb{E} \left( \left|\left\langle U, X\right\rangle\right|^p\right)}{\mathbb{P}_0\left(\left|\left\langle U, x\right\rangle\right| \ge 1\right)}\,. \label{eq:var-moment-tail} \]
In [Bah22], we proposed \eqref{eq:var-moment-tail} and its underlying idea as explained above, and showed that, despite being simple, it reproduces highly non-trivial tail bounds in examples that previously analyzed using more sophisticated techniques. As an example, in [Bah22, Theorem 2] we reproduced a dimension-free matrix concentration inequality due to Zhivotovskiy [Zhi24, Theorem 1] who uses the PAC-Bayesian argument (with an ingenious choice of the “posterior” distributions), and extends a similar result for sample covariance operators due to Koltchinskii and Lounici [KL17, Theorem 9] who in turn relied on a generic chaining argument.
Bibliography
[Bah25] S. Bahmani, “Variational tail bounds for norms of random vectors and matrices,” arXiv preprint, 2025.
[Zhi24] N. Zhivotovskiy, “Dimension-free bounds for sums of independent matrices and simple tensors via the variational principle”, Electron. J. Probab. 29: 1–28, 2024.
[KL17] V. Koltchinskii, K. Lounici, “Concentration inequalities and moment bounds for sample covariance operators”, Bernoulli 23(1): 110–133, 2017.
Let \(\mathbb{V}\) be the vector space of functions from \(\mathcal X\) to \(\mathbb{R}\) whose moment generating function is bounded in a neighborhood of the origin. Given a function class \(\mathcal{F}\subset \mathbb{V}\), and i.i.d. samples \(X_1,\dotsc,X_n\) of a random variable \(X\in \mathcal{X}\) such that \(\mathbb E f(X)=0\) for all \(f\in \mathcal{F}\), a fundamental problem in the theory of empirical processes is finding tail bounds for the empirical average \(\mathbb{E}_n f(X) \overset{\scriptscriptstyle\textrm{def}}{=} n^{-1}\sum_{i=1}^n f(X_i)\) that hold simultaneously for all \(f \in \mathcal{F}\). Such tail bounds are predominantly expressed as the sum of a term depending on some notion of “complexity” of the function class (Rademacher complexity, V.C. dimension, etc), and a term that depends on the worst-case deviations of the random variables \(f(X)\) as \(f\) varies in the function class.
For any single function \(f\in \mathbb{V}\), the Chernoff bound provides a concentration inequality that depends on the deviations of the considered function \(f\) as captured by the corresponding cumulant-generating function. Howerver, the tail bounds that hold uniformly for all functions of a function class, say \(\mathcal{F}\), are commonly expressed in terms of the worst-case deviations across \(\mathcal{F}\). It is therefore natural to ask, if we can derive tail bounds for \(\mathbb{E}_n f\) that hold for every function in \(\mathcal{F}\), but consider instance-dependent deviations rather than the worst-case deviations.
In the case of Gaussian random variables and the class of linear functions defined by inner products with unit vectors, Lugosi and Mendelson [LM20] derived such instance-dependent tail bounds, as a benchmark for their main goal of designing a direction-dependent robust mean estimator. They showed that it can provide significantly sharper inequalities compared to the conventional tail bounds. In [Bah22], we showed how to derive instance-dependent tail bounds in a much broader setting described as follows. For \(r\ge 0\), we define the functionals \(T_r\colon \mathbb{V}\to \mathbb{R}_{\ge 0}\) as \[T_r(f) \overset{\scriptscriptstyle\textrm{def}}{=}\inf_{\lambda>0}\frac{r+\log\mathbb{E} e^{\lambda f\left(X\right)}}{\lambda}\nonumber\,,\] and show that \[\overline{T}_r(f) = \max\{T_r(f), T_r(-f)\}\,\nonumber\] defines a seminorm over \(\mathbb{V}\). Furthermore, let \(A\) be a map from \(\mathcal{F}\) to at most \(e^k\) functions in \(\mathcal{F}\), such that \(A[0]=0\) and \[T_r(A[f]) \le T_r(f)\nonumber\,,\] for every \(f\in \mathcal{F}\). Define the set \[\mathcal{A} = \lbrace f - A[f]\colon\, f\in \mathcal{F}\rbrace\,.\nonumber\] In [Bah22] we define a certain generalization of Talagrand's (truncated) \(\gamma_\alpha\) functionals as \[\gamma(\mathcal A;r, \underline{\ell}) = \inf_{(\mathcal A_i)_{i\ge 0}}\sup_{a\in \mathcal A} \sum_{\ell \ge \underline{\ell}} \overline{T}_{r + (r+1/n)2^{\ell-\underline{\ell}}}(a - \mathcal A_\ell)\,,\] where the infimum is taken over an increasing admissible sequence of subsets of \(\mathcal A\) and \(\overline{T}_s(a -\mathcal A_\ell)\) denotes the distance of \(a\in \mathcal A\) from \(\mathcal A_\ell\) as measured by \(\overline{T}_s(\cdot)\). We show that with probability at least \(1-2e^{-nr}\), for every \(f\in \mathcal{F}\) we have \[\mathbb{E}_n f(X) - \overline{T}_{r+k/n}(f)\le 2\gamma(\mathcal{A};\, r, \lfloor \log_2(nr/5) \rfloor) +\mathrm{rad}_{2r+1/n}(\mathcal{A})\,, \nonumber\] where \(\mathrm{rad}_s\left(\mathcal{A}\right)\) is the radius of \(\mathcal{A}\) as measured by \(\overline{T}_s(\cdot)\).
Bibliography
[LM20] G. Lugosi and S. Mendelson, “Multivariate mean estimation with direction-dependent accuracy,” J. Eur. Math. Soc. 26(6): 2211–2247, 2024.
[Bah22] S. Bahmani, “Instance-dependent uniform tail bounds for empirical processes,” arXiv preprint, 2022.
Estimation of mean of a random variable is a fundamental problem of statistics since many estimation problems such as density estimation, regression, \(\ldots\), can be posed as mean estimation. The most benign and pedagogical example one can imagine is perhaps estimation of the mean of a random Gaussian vector in \(\mathbb{R}^d\), from a finite number of i.i.d. samples. The sample mean, the average of the observed samples, turns out to achieve the best possible confidence interval in the Gaussian setting. Unfortunately, the sample mean loses its glory as soon as we start dealing with heavy-tailed distributions or adversarially manipulated samples. In such unfavorable scenarios, the fundamental questions are then, “How close can we get to the ideal confidence intervals?, With what kind of mean estimators?, and Whether such estimators are computationally tractable?” There has been great strides in this line of research recently; we highly recommend the great survey papers [LM19] and [DK19] for more details on these recent advances as well as the historical background.
In [Bah20], we propose a competing mean estimator (in a separable Banach space) based on empirical characteristic function. For the sake of simpler exposition, we explain the ideas in the usual Euclidean setting. Given i.i.d. copies \(X_1,\dotsc,X_n\) of a random vector \(X\) in \(\mathbb{R}^d\), we want to estimate the mean of \(X\) denoted, by \(\mu_\star\) with error measured in the \(\ell_2\)-norm. The only restriction on the law of \(X\) is that the corresponding covariance matrix, \(\varSigma_\star\), exists and is bounded. Furthermore, we may face strong contamination of the samples, where for some \(\eta\in(0,1/2)\), an adversary may arbitrarily manipulate up to \(\eta n\) samples.
With the empirical characteristic function denoted by \[ \varphi_n(w) = \frac{1}{n}\sum_{i=1}^n \exp(\langle w, X_i\rangle)\nonumber\,, \] our proposed estimator is \[\widehat{\mu} \in \operatorname*{argmin}_\mu \max_{w\,:\,{\| w\|}\le r_n} \langle w, \mu\rangle - \mathrm{Im}(\varphi_n(w))\,,\nonumber\] for appropriately chosen radius \(r_n>0\). In particular, in the non-adversarial setting, we show that by choosing \(r_n = \frac{22\log(1/\delta)}{n\epsilon}\), with probability at least \(1-\delta\) the estimator achieves the bound \(\|\hat{\mu}-\mu_\star\|\le \epsilon\), if the prescribed \(\epsilon\) obeys \[\begin{align*} \epsilon & \ge \max\Big\{96\sqrt{\frac{\mathrm{tr}(\varSigma_\star)}{n}}+12\sqrt{\frac{\|\varSigma_\star\|_{\mathrm{op}}\log(1/\delta)}{n}},\\ & \hphantom{\max\Big\{ }\quad9{\left(\frac{\log(1/\delta)}{n}\right)}^{2/3}\|\mu_\star\|\Big\}\,.\end{align*}\] We also prove a similar statement in the adversarial setting. Basically, the effect of contamination in terms of the contamination parameter \(\eta\in(0,1/2)\) is an additional term of order \(\sqrt{\eta\|\varSigma_\star\|_{\mathrm{op}}}\) that appears in the confidence interval. Both of the derived confidence intervals have an undesirable dependence on \(\|\mu_\star\|\) that, even though vanishing at a rate \(n^{-2/3}\), prevents achieving the ideal purely sub-Gaussian rate. In [Bah20] we discuss ways to diminish this nuisance. Furthermore, we discuss how the estimator can be made oblivious to the accuracy level \(\epsilon\). The main drawback of the estimator is that, superficially, it does not appear to be computationally tractable.
Bibliography
[Bah20] S. Bahmani, “Nearly optimal robust mean estimation via empirical characteristic function,” arXiv preprint, 2020.
[LM19] G. Lugosi and S. Mendelson, “Mean estimation and regression under heavy-tailed distributions: A survey,” Foundations of Computational Mathematics, 19(5):1145–1190, 2019.
[DK8] I. Diakonikolas and D. Kane, “Recent advances in algorithmic high-dimensional robust statistics,” arXiv preprint, 2019.
Bibliography
[BR19] S. Bahmani and J. Romberg, “Convex programming for estimation in nonlinear recurrent models,” 2019.
Bibliography
[Bah18] S. Bahmani, “Estimation from nonlinear observations via convex programming, with application to bilinear regression,” Elect. J. Statistics, 13(1): 1978–2011, 2019.
Problem statement
Consider the problem of estimating an \(N\)-dimensional signal \(\boldsymbol{x}_\star\) from observations of the form \begin{align} y_m & = f_m(\boldsymbol{x}_\star) + \xi_m\,, & m=1,2,\dotsc,M\,, \label{cvxreg} \end{align} where the functions \(f_m\) are i.i.d. copies of a randomly drawn convex function \(f\), and the noise terms are represented by \(\xi_m\). Despite the convexity assumption on the functions \(f_m\), the observation model \eqref{cvxreg} is quite general and includes many standard statistical models as the special case including generalized linear models and single hidden layer neural nets. I addressed the general regression problem \eqref{cvxreg} in [BR17b] building upon ideas I developed in [BR17a] to address a special case often known as phase retrieval. It is more illustrative to begin my explanation with this special case as well.
A new approach to phase retrieval
The problem of phase retrieval appears in areas such as imaging and optics where the sensors often measure only intensities; the sign- (or phase-) information is generally lost. The observation model in the phase retrieval problem, assuming no noise in the measurements, can be abstracted as the system of quadratic equations \begin{equation*} \begin{aligned} y_1 & = |\boldsymbol{a}_1^*\boldsymbol{x}_\star|^2\\ y_2 & = |\boldsymbol{a}_2^*\boldsymbol{x}_\star|^2\\ \vdots & \qquad\vdots\\ y_M & = |\boldsymbol{a}_M^*\boldsymbol{x}_\star|^2\,, \end{aligned} \end{equation*} for \(\boldsymbol{x}_\star\in\mathbb{C}^N\). Clearly, this model corresponds to \eqref{cvxreg} with \(f_m(\boldsymbol{x}) = |\boldsymbol{a}_m^*\boldsymbol{x}|^2\) for i.i.d. draws of \(\boldsymbol{a}_m\), and \(\xi_m=0\). In [BR17a], I formulated a new estimator for this problem as the convex program \begin{equation} \begin{aligned} \operatorname*{argmax}_{\boldsymbol{x}}\ & \mathrm{Re}(\boldsymbol{a}_0^*\boldsymbol{x}) & \\ \text{subject to}\ & |\boldsymbol{a}_m^*\boldsymbol{x}|^2 \le y_m, & m=1,2,\dotsc,M\,, \end{aligned} \label{linmax} \end{equation} where \(\boldsymbol{a}_0\) denotes an “anchor vector” that obeys \begin{equation*} |\boldsymbol{a}_0^*\boldsymbol{x}_\star| \ge \delta \left\lVert\boldsymbol{a}_0\right\rVert_2 \left\lVert\boldsymbol{x}_\star\right\rVert_2\,, \end{equation*} for some absolute constant \(\delta \in (0,1]\). The anchor vector can be constructed from random observations similar to initializations in some non-convex methods (e.g., Wirtinger Flow); the details can be found in the paper. Here, I explain the geometric intuition behind \eqref{linmax} in the case of real-valued variables for clarity. As illustrated in Figure 1, each of the constraints in \eqref{linmax} form a slab of feasible points, whose intersection is a convex polytope \(\mathcal{K}\). Clearly, \(\boldsymbol{x}_\star\) is an extreme point of \(\mathcal{K}\). The solution to \eqref{linmax} is also always an extreme point of \(\mathcal{K}\), since it is a solution to linear maximization over the convex body \(\mathcal{K}\). The key observation is that if the anchor vector \(\boldsymbol{a}_0\) has a non-trivial component in the direction of \(\boldsymbol{x}_\star\), we can expect that the extreme point found by \eqref{linmax} coincides with \(\boldsymbol{x}_\star\).
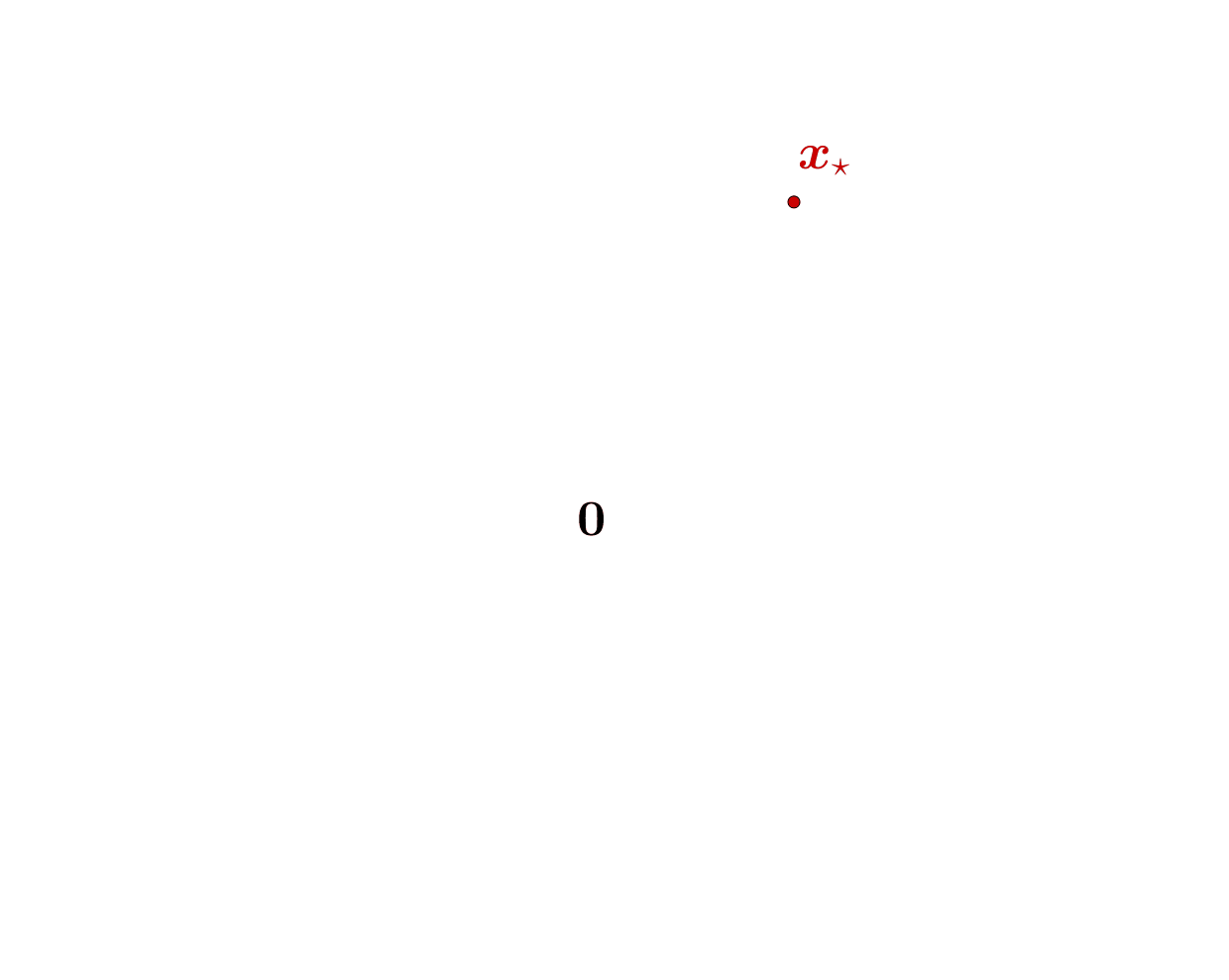
Using classic results from statistical learning theory, I showed that with high probability \begin{equation*} M = C_\delta N \end{equation*} independent random measurements suffice to recover \(\boldsymbol{x}_\star\) using \eqref{linmax}, with \(C_\delta\) being an absolute constant depending only on \(\delta\). Robustness under specific noise models is also addressed in the paper.
Why does \eqref{linmax} matter?
Previous convex relaxations for phase retrieval (e.g., PhaseLift, and PhaseCut) were based on the idea of lifting and semidefinite programming (SDP). While lifting-based methods are technically computationally tractable, their dependence on SDP prohibits their scalability. In contrast, \eqref{linmax} operates in the natural domain of the problem and competes with non-convex methods for phase retrieval (e.g., Wirtinger Flow). It also benefits from versatility, flexibility, and robustness that is associated with convex programming. More importantly, as discussed below, the principles used in formulation and analysis of \eqref{linmax} apply in a more general setting.
What about the general case \eqref{cvxreg}?
In [BR17b], I proposed the convex program \begin{equation} \begin{aligned} \operatorname*{argmax}_{\boldsymbol{x}}\ & \langle \boldsymbol{a}_0,\boldsymbol{x}\rangle \\ \text{subject to}\ & \sum_{m=1}^M \max\{f_m(\boldsymbol{x})-y_m, 0\} \le \varepsilon\, , \end{aligned} \label{anchored_reg} \end{equation} where \(\boldsymbol{a}_0\) is an “anchor vector” that obeys \begin{equation*} \langle\boldsymbol{a}_0,\boldsymbol{x}_\star\rangle \ge \delta \left\lVert\boldsymbol{a}_0\right\rVert_2\left\lVert\boldsymbol{x}_\star\right\rVert_2\,, \end{equation*} as an estimator for the general regression problem \eqref{cvxreg}. Some schemes for constructing the anchor from the measurements are described in the paper, but we omit the discussion for brevity. Furthermore, to avoid technical details, here I state the main result of the paper (i.e., Theorem 2.1) on the sample complexity of \eqref{anchored_reg} in an informal way. In the proved bound, there are two important quantities. The first quantity, \(\mathfrak{C}_M(\mathcal{A}_\delta)\), measures the “size” of a set \(\mathcal{A}_\delta\) that depends only on \(\boldsymbol{x}_\star\) and \(\delta\), with respect to the randomness in the gradients \(\nabla f_m(\boldsymbol{x}_\star)\). The second quantity, \(p_\tau(\mathcal{A}_\delta)\), is some measure of the “eccentricity” of the random vector \(\nabla f_m(\boldsymbol{x}_\star)\) with respect to the set \(\mathcal{A}_\delta\) in terms of a parameter \(\tau\). Ignoring some details, the result established in [BR17b] basically states that \begin{equation*} M \gtrsim \left(\frac{\mathfrak{C}_M(\mathcal{A}_\delta)}{\tau p_\tau(\mathcal{A}_\delta)}\right)^2\,, \end{equation*} measurements are sufficient to guarantee that \eqref{anchored_reg} yields an accurate estimate.
Bibliography
[BR17a] S. Bahmani and J. Romberg, “Phase retrieval meets statistical learning theory: A flexible convex relaxation,” In Proceedings of the 20th International Conference on Artificial Intelligence and Statistics (AISTATS'17), vol. 54 of Proceedings of Machine Learning Research , pp. 252–260.
[BR17b] S. Bahmani and J. Romberg, “Solving equations of random convex functions via anchored regression,” Foundations of Computational Mathematics, 19(4):813–841, 2019.
An important characteristic of networks in many applications is their connectivity which is often a
crucial factor in the performance of the network. An interesting and important problem is then to measure
robustness of the connectivity under some form of perturbation of the network. Site percolation, or
simply random removal of nodes as illustrated in Figure 2, is one of these
perturbation models that is studied in mathematics and statistical physics.
Algebraic connectivity of a graph is a an analytical measure of connectivity that is also related to the conductance of the graph through the Cheeger's inequality. Formally, the algebraic connectivity of a graph with the adjacency matrix \(\boldsymbol{A}\) can be defined as the second smallest eigenvalue of the graph Laplacian \(\boldsymbol{L} = \boldsymbol{D} - \boldsymbol{A}\) where \(\boldsymbol{D}=\mathrm{diag}(\boldsymbol{A}\boldsymbol{1})\) is the diagonal matrix of the vertex degrees. In [BRT18], using tools from random matrix theory I derived a lower bound for algebraic connectivity of a graph that survives from a generally non-homogeneous site percolation. In the special case of homogeneous site percolation over a certain class of regular graphs, our analytical result virtually coincides with the state-of-the-art that is established using refined combinatorial arguments.
Bibliography
[BRT18] S. Bahmani, J. Romberg, and P. Tetali, “Algebraic connectivity under site percolation in finite weighted graphs,” IEEE Trans. Network Science and Engineering, 5(2):86–91, 2018.
Publications
- In Review/Revision
- Journal Paper
- Conference Paper
- Tech. Report/Unpublished
In Review/Revision
- S. Bahmani, “Variational tail bounds for norms of random vectors
and matrices,” 2025.
arXiv - S. Bahmani, “A fundamental accuracy—robustness trade-off in
regression and classification,” 2024.
arXiv - S. Bahmani, “Instance-dependent uniform tail bounds for
empirical processes,” 2022.
arXiv
2024
- S. Kim, S. Bahmani, K. Lee, “Max-linear regression by convex
programming,” IEEE Trans. Info. Theory, 2024.
IEEEXplorearXiv
2021
- S. Bahmani, K. Lee, “Low-rank matrix estimation from rank-one
projections by unlifted convex optimization”, SIAM J. on Matrix Analysis
and Applications, 2021.
arXivSIAM - S. Bahmani, “Nearly optimal robust mean estimation via empirical characteristic function,” Bernoulli, 27(3): 2139–2158, 2021.arXivProj. Euclid
- B. Ancelin, S. Bahmani, J. Romberg, “Decentralized
feature-distributed optimization for generalized linear models,”, 2021.
arXiv
2020
- S. Bahmani, J. Romberg, “Convex programming for estimation in nonlinear recurrent models,” Journal of Machine Learning Research, (235):1–20, 2020.arXivJMLRCode
- K. Lee, S. Bahmani, J. Romberg, Y. Eldar, “Phase retrieval of low-rank matrices by anchored regression,” Information and Inference: A Journal of the IMA, 2020.arXivOxford Journals
2019
- S. Bahmani, “Estimation from nonlinear observations via convex programming, with application to bilinear regression,” Electronic J. of Statistics, 13(1): 1978–2011. 2019.arXivProj. Euclid
2018
- S. Bahmani and J. Romberg, “Solving equations of random convex functions via anchored regression,” Foundations of Computational Mathematics, 19(4):813–841, 2019.arXivSpringer
- S. Bahmani, J. Romberg, P. Tetali, “Algebraic connectivity under site percolation in finite weighted graphs,” IEEE Trans. on Network Science and Engineering, 5(2):86–91, 2018. arXivIEEEXplore
2017
- S. Bahmani and J. Romberg, “A
flexible convex relaxation for phase retrieval,” Electronic Journal of Statistics,
11(2):5254–5281, 2017. (This is
an extended version of the AISTATS’17 paper.)
Proj. Euclid - S. Bahmani and J. Romberg, “Phase retrieval meets statistical learning theory: A flexible convex relaxation,” In Proceedings of the 20th International Conference on Artificial Intelligence and Statistics (AISTATS'17), vol. 54 of Proceedings of Machine Learning Research , pp. 252–260. (Best paper award) arXivPMLR
2016
- S. Bahmani and J. Romberg, “Near-optimal estimation of simultaneously sparse and low-rank matrices from nested linear measurements,” Information and Inference: A Journal of the IMA 5(3):331–351, 2016.arXivOxford Journals
- S. Bahmani, P. Boufounos, and B. Raj, “Learning model-based sparsity via projected gradient descent,” IEEE Trans. Info. Theory, 62(4):2092–2099, 2016.arXivIEEEXplore
2015
- S. Bahmani and J. Romberg, “Sketching for simultaneously sparse and low-rank covariance matrices,” in Computational Advances in Multi-Sensor Adaptive Processing (CAMSAP'15), IEEE 6th International Workshop on, pp. 357–360, Cancun, Mexico, Dec. 2015.arXivIEEEXplore
- S. Bahmani and J. Romberg, “Efficient compressive phase retrieval with constrained sensing vectors,” in Advances in Neural Information Processing Systems (NIPS'15), vol. 28, pp. 523–531, Montréal, Canada, Dec. 2015.arXivNIPS
- S. Bahmani and J. Romberg, “Lifting for blind deconvolution in random mask imaging: Identifiability and convex relaxation,” SIAM Journal on Imaging Sciences, 8(4):2203–2238, 2015.arXivSIAM
- S. Bahmani and J. Romberg, “Compressive deconvolution in random mask imaging,” IEEE Trans. on Computational Imaging, 1(4):236–246, 2015.arXivIEEEXplore
2013
- S. Bahmani, B. Raj, and P. T. Boufounos, “Greedy sparsity-constrained optimization,” Journal of Machine Learning Research, 14(3):807–841, 2013.arXivJMLRCode
- S. Bahmani, P. Boufounos, and B. Raj, “Robust 1-bit compressive sensing via gradient support pursuit,” Apr. 2013.arXiv
2012
- S. Bahmani, B. Raj, “A unifying analysis of projected gradient descent for \(\ell_p\)-constrained least squares,” Applied and Computational Harmonic Analysis, 34(3):366–378, 2012.arXivElsevier
2011
- S. Bahmani, P. Boufonos, and B. Raj, “Greedy sparsity-constrained optimization,” in Conf. Record of the 45th Asilomar Conference on Signals, Systems, and Computers (ASILOMAR'11), pp. 1148–1152, Pacific Grove, CA, Nov. 2011. IEEEXploreSlidesCode
2010
- S. Bahmani, I. Bajić, and A. HajShirmohammadi, “Joint decoding of unequally protected JPEG2000 images and Reed-Solomon codes,” IEEE Trans. Image Processing, 19(10):2693–2704, Oct. 2010.IEEEXplore
2009
- S. Bahmani, I. Bajić, and A. HajShirmohammadi, “Improved joint source channel decoding of JPEG2000 images and Reed-Solomon codes,” Proc. IEEE ICC'09, Dresden, Germany, Jun. 2009.IEEEXplore
2008
- S. Bahmani, I. Bajić, A. HajShirmohammadi, “Joint source channel decoding of JPEG2000 images with unequal loss protection,” Proc. IEEE ICASSP'08, pp. 1365–1368, Las Vegas, NV, Mar. 2008.IEEEXplore
Thesis
- S. Bahmani, Algorithms for sparsity-constrained optimization, PhD dissertation, Department of Electrical & Computer Engineernig, Carnegie Mellon University, Pittsburgh, PA, Feb. 2013.PDF